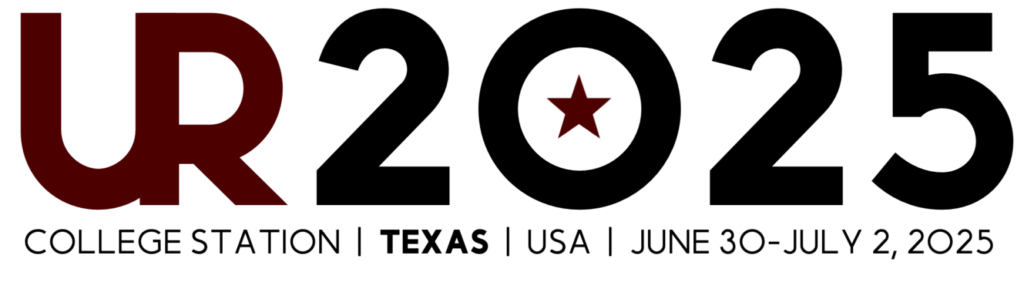
* The registration fee for workshops only is $100 (Regular)/$50 (Student).
Overview
This workshop explores the transformative role of virtual environments and synthetic data generation (SDG) in advancing robotic training. As interest in capable robots, including humanoids, grows, the need for efficient and robust training methods becomes critical. Gathering diverse, large-scale real-world datasets is often costly and time-consuming. Synthetic data, generated within physically accurate digital twins and simulations, offers a powerful alternative to train and validate AI models before real-world deployment. This workshop will delve into simulations, high-fidelity modeling, and the creation of synthetic datasets tailored for robotics.
Schedule
June 30, Monday
Schedule | Breakout Room A |
13:00 – 18:00 | Workshop 1 |
Each presentation segment is allocated 30 minutes, allowing for comprehensive coverage of key topics while maintaining an engaging pace. This format ensures we have adequate time for both in-depth technical discussions and interactive Q&A sessions. After you have successfully registered for UR 2025, you can upload your presentation file (PDF/PPTX) by following the link below. The slides could be updated later. Please contact Dr. Jian Tao (jtao [at] tamu [dot] edu) if you have any questions.
Key Themes and Topics
- Synthetic Data Generation Techniques. Methods for creating scalable and diverse datasets using 3D models, generative AI, and physics-based simulations.
- Action Recognition with Synthetic Data. Training machine-learning models to recognize human actions using synthetic datasets for applications like smart homes, construction safety, and healthcare robotics.
- Digital Twins in Robotics. Utilizing digital twins as interactive virtual replicas to simulate real-world robotic environments for training and testing.
- High-Fidelity Modeling. Developing photorealistic environments and sensor simulations (e.g., LiDAR, RGB-D cameras) to bridge the gap between virtual and real-world conditions.
- Sim2Real Transfer Learning. Addressing challenges in transferring knowledge from synthetic environments to real-world applications.
- Ethical and Privacy Considerations. Discussing how synthetic data can eliminate privacy violations, contextual biases, and copyright issues associated with real datasets.
- Scalability of Synthetic Datasets. Techniques for generating large-scale datasets that rival traditional annotated datasets in size and diversity.
- Future Directions in Synthetic Data Research. Combining synthetic data generation with advanced video platforms to create more realistic datasets and exploring how models trained on synthetic data learn differently compared to real-world data.
Why Attend?
Synthetic data is proving essential for teaching robots complex, humanlike behaviors. Models trained using synthetic data have demonstrated performance comparable or even superior to those trained on real data1. This workshop provides a forum to:
- Learn about the latest advancements in virtual environments and SDG for robotics.
- Understand how SDG can overcome the limitations of real-world data collection.
- Explore methods to improve robot capabilities, adaptability, and accuracy through simulation-based training.
- Connect with researchers and practitioners focused on the future of robotic learning and physical AI.
Organizers

Dr. Jong-in Lee is an Assistant Professor in the School of Visualization at Texas A&M University’s College of PVFA. Specializing in Human-Computer Interaction and 3D User Interface research, he develops novel interaction techniques for spatial computing environments. Dr. Lee obtained his PhD in Interactive Arts and Technology from Simon Fraser University, a Master’s in Culture Technology from KAIST, and Bachelor’s degrees in Computer Science and Film/TV/Multimedia from Sung Kyun Kwan University. His research focuses on creating advanced 3D navigation techniques that enhance spatial awareness in immersive environments, while also developing high-fidelity prototyping tools that streamline XR interface design. This work bridges traditional design workflows with the unique challenges of spatial computing, enabling more intuitive and effective XR experiences.
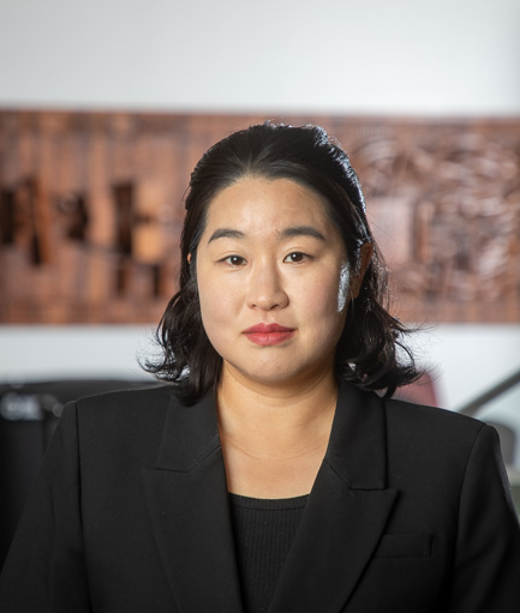
Dr. Jimin Rhim conducts interdisciplinary research on the design of emerging technologies, with a particular focus on interactive social agents, including robotics and artificial intelligence. Her work aims to develop human-centered systems that advance societal well-being and innovation. Dr. Rhim received her Ph.D. from the Graduate School of Culture and Technology at the Korea Advanced Institute of Science and Technology (KAIST), where her doctoral dissertation was recognized as one of KAIST’s top research achievements in 2019. She is currently an Instructional Assistant Professor at Texas A&M University. Prior to her current role, Dr. Rhim held several postdoctoral research positions across multiple disciplines, including the School of Computing Science at Simon Fraser University, the Department of Electrical and Computer Engineering at McGill University, and the School of Engineering Design and Teaching Innovation at the University of Ottawa. She also served as a Research Associate in the Faculty of Health Sciences at Simon Fraser University.
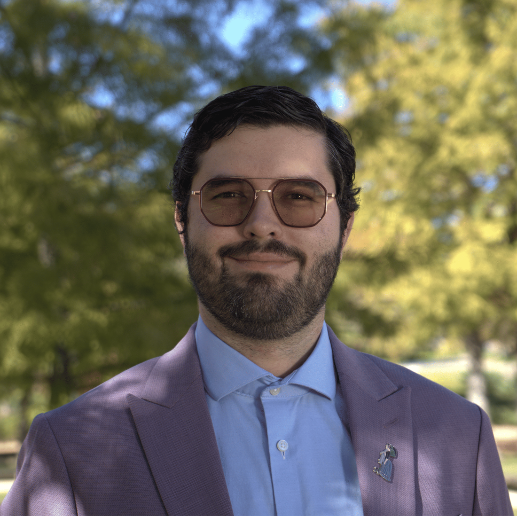
Dr. Edgar J. Rojas-Muñoz joined Texas A&M as an Assistant Professor in Fall 2022. He received his PhD in Industrial Engineering from Purdue University in 2020, and his Licenciatura in Computer Engineering from the Costa Rica Institute of Technology in 2016. His work is centered on Mixed Reality technologies for the Social Good. He believes these technologies are meant to become the ultimate human-computer interface, and therefore focuses his work on addressing the technical challenges, user considerations, and overall applications that are required to help this field achieve its true potential. Dr. Rojas-Muñoz is the director of the Laboratory for Extended and Mixed User Realities (LEMUR). His research direction is focused on four main directions: MR for safety and situation awareness, MR for remote education, MR for theater and storytelling, and MR for accessibility and inclusion.

Dr. Jian Tao is an Assistant Professor from the Section of Visual Computing & Computational Media in the College of Performance, Visualization & Fine Arts at Texas A&M University. He is also the Director of the Digital Twin Lab and the Assistant Director for Project Development at the Texas A&M Institute of Data Science. Tao also holds courtesy appointments at the Department of Electrical & Computer Engineering, the Department of Nuclear Engineering, and the Department of Multidisciplinary Engineering in the College of Engineering at Texas A&M University. His research interests include digital twin, numerical modeling, machine learning, data analytics, distributed computing, visualization, and workflow management.
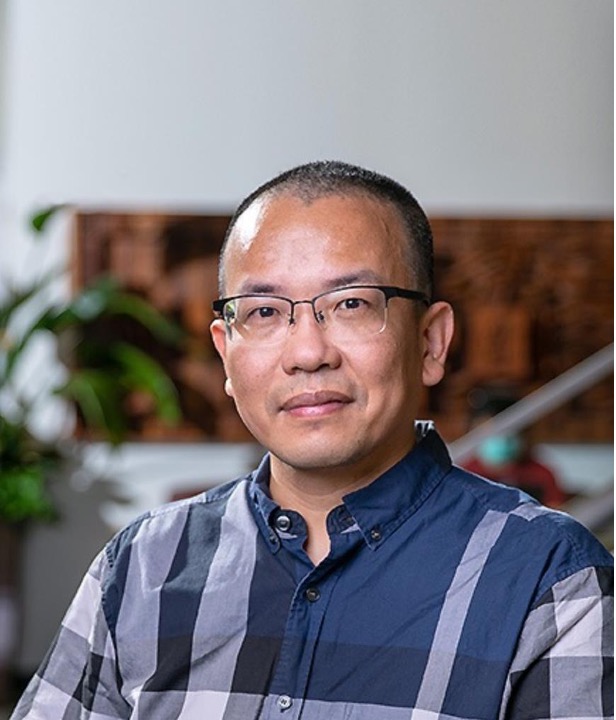
Dr. Xinyue Ye is the Harold Adams Endowed Professor in Urban Informatics and Stellar Faculty Provost Target Hire at Texas A&M University (TAMU). He serves as the Faculty Fellow (Strategic Initiatives and Partnerships) for The Division of Research at TAMU. Directing the Center for Geospatial Sciences, Applications, and Technology, Dr. Ye integrates computational social science, urban data science, and geospatial artificial intelligence (GeoAI) to address issues ranging from infrastructure resilience and extreme weather preparedness to urban revitalization and community perceptions. Supported by 13 federal agencies and numerous industry partners, his team underscores the dynamic interplay between technology, policy, and human behavior in shaping sustainable and livable cities. His current research is centered on urban digital twins and precision public health, emphasizing real-time 3D modeling and AI-enabled participatory planning. Additionally, his work focuses on urban environmental science, with an emphasis on understanding the impacts of weather extremes on the built environment and human mobility. Due to his innovative research integrating computer science, geography, and planning, Dr. Ye is the first scholar to be elected under the Early/Mid-Career category for a Fellow of the American Association of Geographers (AAG, 2022-). Additionally, Dr. Ye is a Fellow of the Royal Geographical Society (2022-). He received the AAG Distinguished Scholar Award for 2025, achieving this distinction in the shortest interval in AAG history, earning the award just 15 years after completing a Ph.D. in 2010. He has been among the world’s top 2% scientists based on both career-long and year-specific citations by Stanford University since 2020.
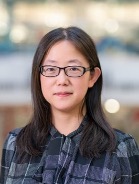
Dr. Minghui Zheng received the B.S. degree in engineering mechanics in 2008 and M.S. degree in control science and engineering in 2011, both from Beihang University, China, and the Ph.D. degree in mechanical engineering from University of California, Berkeley, CA, USA, in 2017. She is currently an Associate Professor of the J. Mike Walker ’66 Department of Mechanical Engineering, Texas A&M University, TX, USA. Before that, she was a faculty member at the University at Buffalo, NY, USA. Her research interest lies in learning, planning, and control with applications to several areas that are of vital importance to manufacturing and robotics. Dr. Zheng was the recipient of the NSF CAREER Award in 2021.